TL; DR: Not Onboarding But Integration
Stop treating AI as a team member to “onboard.” Instead, give it just enough context for specific tasks, connect it to your existing artifacts, and create clear boundaries through team agreements. This lightweight, modular approach of contextual AI integration delivers immediate value without unrealistic expectations, letting AI enhance your team’s capabilities without pretending it’s human.
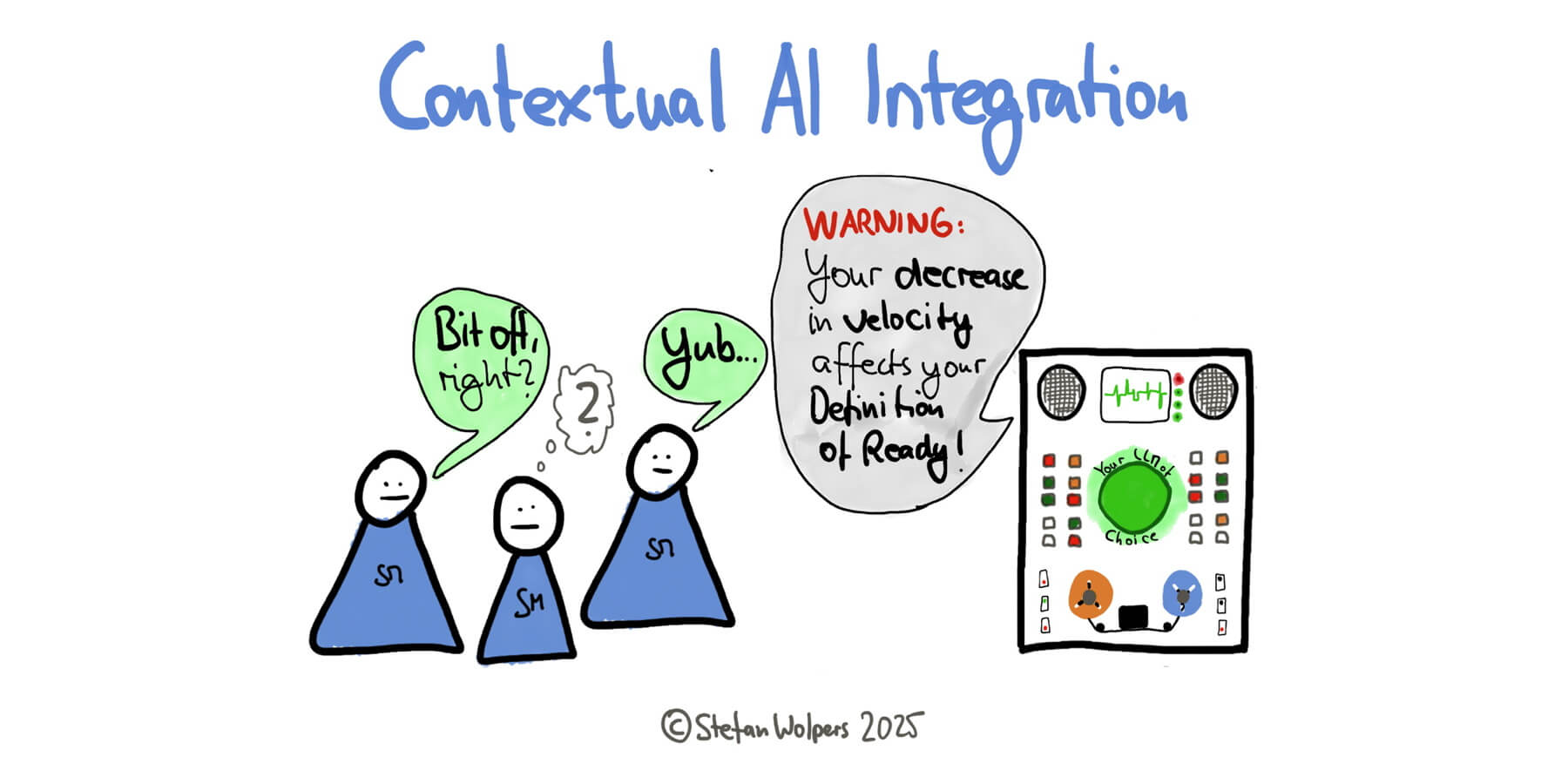
Contextual AI Integration for Agile Product Teams
Imagine this scenario: An empowered product team implements an AI assistant to help with feature prioritization and customer insights. Six weeks later, the Product Owner finds its ranking suggestions use irrelevant criteria, product designers notice it ignores established design patterns, and developers see it making technically sound suggestions that are misaligned with their architecture. Despite everyone using the same AI tool, it doesn’t understand how the product team actually works.
This scenario represents a not-uncommon challenge in agile product organizations. Teams operating within a product operating model—cross-functional, empowered teams responsible for discovering and delivering valuable products—find that generic AI tools struggle to integrate with their well-established ways of working.
The problem isn’t a lack of AI capabilities, but a fundamental misalignment between how AI systems operate and how agile product teams work. Many organizations mistakenly treat AI implementation as “onboarding a new team member,” ignoring the fundamental differences between human cognition and artificial intelligence.
This article introduces Contextual AI Integration—a lightweight, modular approach for incorporating AI into agile product teams that recognizes the unique characteristics of both AI systems and agile environments.
AI Is Not a Team Member: Understanding the Fundamental Differences
Before discussing contextual AI integration approaches, we must recognize why treating AI as a new team member to be “onboarded” creates unrealistic expectations:
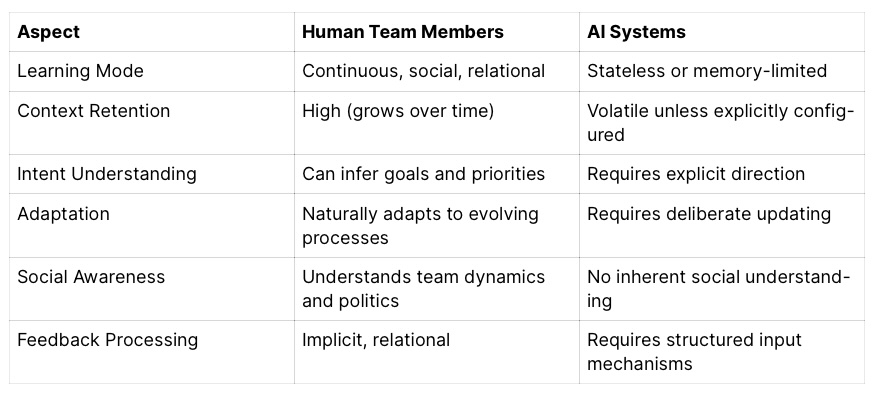
Experience shows that teams often allocate significant time teaching AI systems about their entire product development process. However, providing specific, focused context for particular tasks often works better, tied directly to existing artifacts like user stories and acceptance criteria.
The Integration Challenge: Why AI Systems Struggle in Agile Environments
Empowered product teams face specific obstacles when integrating AI into their processes:
Context Poverty Challenge: AI systems lack understanding of team-specific language, priorities, and values. A banking product team may find their AI assistant consistently misinterpreted their user story discussions because terms like “account” have different meanings across their product ecosystem, and the AI cannot distinguish these nuances without specific guidance.
Alignment Gaps Problem: AI tends to optimize for whatever metrics it can most easily measure, often missing the team’s actual priorities. A healthcare product team may see their AI maximize appointment density while ignoring the unpredictable timing needs of different appointment types. The AI may create technically “efficient” schedules that collapse when a single appointment runs long—missing the team’s hard-earned knowledge about reasonable buffer times.
Resistance Pattern: Team members reject AI that doesn’t respect their implicit working agreements. A development team may abandon an AI code reviewer that makes technically correct but contextually inappropriate suggestions, showing no awareness of the team’s release and DevOps practices.
Knowledge Silos Issue: Critical product knowledge often exists as tacit understanding rather than documentation. In manufacturing, an AI quality control system might repeatedly misclassify acceptable product variations because the tolerances documented in specifications differ from what experienced floor managers actually accept in practice—knowledge that exists in the team but isn’t captured in the training data.
Knowledge Currency Problem: Product development inherently involves continuous evolution in processes, tools, and practices. Without a mechanism to continuously update the AI’s understanding, its recommendations become increasingly misaligned with how the team works. What starts as minor misalignment grows into significant friction as the AI continues operating with outdated assumptions about processes, policies, and tools. Without deliberate attention to maintaining knowledge currency, even initially well-integrated AI systems gradually lose relevance as the team and product evolve.
Contextual AI Integration: A Modular, Artifact-Connected Approach
Rather than attempting to comprehensively “onboard” AI systems, successful agile teams implement contextual integration through these key principles:
1. Situational Role Framing
What It Means: Clearly define what the AI is for in each specific context, rather than treating it as a general team member.
How It Works:
- Define specific AI use cases tied to team roles and events,
- Use role-based prompt engineering (e.g., “You are assisting a Product Owner with backlog refinement…”),
- Connect AI use to specific team activities rather than general assistance.
Implementation Approach:
Consider creating specific AI prompts for different Scrum events. A “Retrospective Assistant” might reference team metrics, agreements, and psychological safety principles. Each would have a narrow, well-defined purpose rather than trying to serve as a general-purpose assistant.
2. Just-In-Time Context Injection
What It Means: Provide AI with minimal viable context exactly when needed, rather than attempting comprehensive knowledge transfer.
How It Works:
- Connect AI to live data sources (JIRA, Confluence, GitHub) for the current context, enabling RAG,
- Use templates with placeholders for dynamic information,
- Create standardized context snippets for common scenarios.
Implementation Approach:
Instead of attempting to teach AI about your entire product strategy, consider creating a simple template like: “Here’s the user story we’re discussing: [STORY]. Here are our acceptance criteria standards: [STANDARDS]. Here are two examples of well-refined stories: [EXAMPLES]. Help us identify missing acceptance criteria for this story.” This focused approach can yield more immediately useful results than providing comprehensive product knowledge and hoping the AI will figure out the rest.
3. Agile Artifact Anchoring
What It Means: Connect AI directly to existing agile artifacts and events rather than creating parallel systems.
How It Works:
- Use the Definition of Done as explicit quality criteria for AI outputs,
- Reference Working Agreements to establish AI interaction boundaries,
- Incorporate team values and principles as evaluation guidelines; for example, the Scrum Guide or Core Principles.
Implementation Approach:
One effective technique is connecting your AI to your Definition of Done and Working Agreements documents as reference material. When developers ask for implementation suggestions, the AI can first check whether its recommendations align with established standards before responding. This approach can significantly reduce the frequency of contextually inappropriate suggestions.
4. AI Working Agreements
What It Means: Explicitly define boundaries for AI use within the team through shared agreements.
How It Works:
- Create transparent guidelines for when and how somebody should use the AI,
- Clarify which decisions remain exclusively human,
- Establish protocols for handling AI suggestions that conflict with team knowledge.
Implementation Approach:
Consider adding an ‘AI Working Agreement’ to your team charter that explicitly states boundaries such as: “AI assists with idea generation and information processing but does not make product decisions. The team must review all AI suggestions before implementation. We commit to tracking which decisions were AI-influenced for learning purposes.” This clarity can help prevent both over-reliance and under-utilization.
Role-Specific Contextual Integration
Different roles on product teams can leverage contextual AI integration in specific ways:
Product Owners
Key Integration Points:
- Connect AI to Product Backlog, user research, and market data,
- Provide context about prioritization frameworks (e.g., WSJF, Kano model),
- Supply examples of well-written Product Backlog items and acceptance criteria.
Example Uses:
- Identifying inconsistencies or gaps in the Product Backlog,
- Generating alternative perspectives on feature descriptions,
- Summarizing customer feedback into actionable insights.
Potential Application:
A Product Owner could connect an AI assistant to their customer feedback database and product analytics. Before prioritization sessions, they might ask the AI to identify patterns across user feedback, usage metrics, and existing Product Backlog items, potentially revealing non-obvious user needs that aren’t explicit in individual feedback items.
Developers
Key Integration Points:
- Connect AI to codebase, architecture documents, and technical standards,
- Provide context about Definition of Done and code review guidelines,
- Supply information about technical debt priorities and (tool) constraints.
Example Uses:
- Suggesting implementation approaches consistent with existing patterns,
- Identifying potential impacts of changes across the codebase,
- Drafting unit tests based on acceptance criteria.
Potential Application:
An AI code assistant could be integrated with architecture decision records (ADRs) and coding standards. Instead of generating generic code, it could suggest implementations that follow established patterns and respect the architectural boundaries of the specific organization.
Product Designers
Key Integration Points:
- Connect AI to the design system, usability heuristics, and accessibility guidelines,
- Provide context about user personas and customer journey maps,
- Supply examples of approved designs and patterns.
Example Uses:
- Generating design alternatives consistent with established patterns,
- Checking designs against accessibility guidelines,
- Suggesting user research questions based on design hypotheses.
Potential Application:
Product designers could provide AI with their design system documentation and examples of past solutions. When exploring new interaction patterns, they could ask it to generate alternatives that maintain consistency with established patterns while solving new problems. This approach could provide a broader exploration space without breaking the established design language.
The Empirical Advantage: Inspect and Adapt AI Integration
The key to successful AI integration is applying Agile’s empirical process control:
Transparency
- Make AI context sources explicitly visible to the team,
- Document which inputs influence AI recommendations,
- Create visibility into how and when AI is used.
Potential Practice:
Teams could maintain a simple “AI context log” showing what information sources their AI tools can access, when they were last updated, and any known gaps or limitations, creating transparency around AI capabilities and limitations.
Inspection
- Regularly review AI contributions for alignment with team needs,
- Analyze patterns of effective and ineffective AI use,
- Evaluate whether AI is supporting or hindering agile values.
Potential Practice:
Teams might conduct bi-weekly “AI alignment checks” to review instances where AI suggestions were particularly helpful or problematic, then adjust their integration approach accordingly.
Adaptation
- Evolve AI context based on empirical evidence,
- Adjust integration patterns based on team feedback,
- Continuously refine the boundaries of effective AI use.
Potential Practice:
A team could track “context misalignments”—instances where AI recommendations missed important context—and use these to build a continuously improving integration approach.
Measuring Success of Contextual AI Integration: Value-Oriented Metrics
Effective AI integration should be measured through outcome-focused metrics rather than adoption statistics:
1. Flow Impact Metrics
- Cycle time for AI-assisted vs. non-assisted work items,
- Frequency of rework on AI-influenced deliverables,
- Team cognitive load assessment (before/after AI integration).
Potential Measurement Approach:
Teams could compare Product Backlog items refined with AI assistance against those refined without AI, measuring metrics like implementation rework and cycle times to quantify impact.
2. Context Alignment Score
- Team ratings of AI recommendation relevance (1-5 scale),
- Frequency of context-related corrections needed,
- Gap between expected and actual AI performance.
Potential Measurement Approach:
For example, developers might track the “contextual relevance” of AI code suggestions on a 1-5 scale. After connecting AI to architecture decision records, teams could measure whether the average relevance score improves.
3. Outcome Contribution
- Impact of AI-assisted work on key product metrics,
- Stakeholder assessment of deliverable quality,
- Innovation rate (new approaches identified).
Potential Measurement Approach:
Product designers could measure the impact of AI-assisted design exploration on final design quality (as rated by users) to determine whether AI assistance contributes to measurable improvements in usability.
Contextual AI Integration Anti-Patterns
Watch for these warning signs of ineffective AI integration:
1. The Comprehensive Onboarding Trap
- Anti-pattern: Spending months trying to teach AI about all aspects of the product and process before allowing any use.
- Solution: Start with minimal viable context for a specific, narrow use case and expand based on empirical results.
2. The AI Team Member Fallacy
- Anti-pattern: Treating AI as if it has human-like understanding, memory, and social awareness.
- Solution: Create clear situational framing for each AI use case and explicit context injection.
3. The Context Staleness Problem
- Anti-pattern: Providing AI with static, point-in-time context that quickly becomes outdated.
- Solution: Implement systematic context refresh mechanisms tied to product and process changes.
4. The Black Box Integration Issue
- Anti-pattern: Treating AI as a magical problem-solver without transparent boundaries.
- Solution: Create explicit AI working agreements and make context sources visible to all team members.
Getting Started: Pragmatic Next Steps
Begin your contextual AI integration journey with these focused activities:
1. Use Case Identification Workshop (1-2 hours)
- Gather your product team to identify specific, high-value activities where AI might help,
- Prioritize based on potential impact and contextual complexity,
- Select one focused use case to start with.
2. Minimal Viable Context Mapping (1 hour)
- For your chosen use case, identify the absolute minimum context needed,
- Document context in simple, structured format (markdown, simple template),
- Identify existing artifacts that could serve as context sources.
3. Integration Experiment Design (1 hour)
- Create a specific hypothesis about how contextual AI will improve the selected activity,
- Design a small experiment with clear success criteria,
- Establish a feedback mechanism to capture team observations.
4. AI Working Agreement Draft (30 minutes)
- Define clear boundaries for initial AI use,
- Clarify how the team will evaluate AI contributions,
- Establish when and how context will be updated.
5. Inspect and Adapt Session (1 hour, after 2-3 weeks)
- Review the results of your integration experiment,
- Identify context gaps and improvement opportunities,
- Adjust your approach based on empirical evidence.
Conclusion: From Tools to Contextual Partners
The path to effective AI integration for agile product teams isn’t through comprehensive “onboarding,” but through thoughtful, contextual integration that respects both AI limitations and agile principles.
By treating AI not as team members but as context-dependent tools, teams can establish integration patterns that deliver immediate value while avoiding unrealistic expectations. The key is connecting AI systems to existing agile artifacts, events, and working agreements—making them contextually aware without pretending they possess human-like understanding.
The breakthrough for many teams comes when they stop trying to make AI understand everything about their product and process. Instead, focusing on giving just the right context for specific tasks, connected to existing ways of working, can deliver immediate value while being much easier to maintain as products evolve.
By applying empirical process control to AI integration itself—making context transparent, regularly inspecting results, and adapting approaches based on evidence—agile teams can transform generic AI capabilities into contextually relevant tools that enhance their ability to deliver valuable products.
🗞 Shall I notify you about articles like this one? Awesome! You can sign up here for the ‘Food for Agile Thought’ newsletter and join 42,000-plus subscribers.