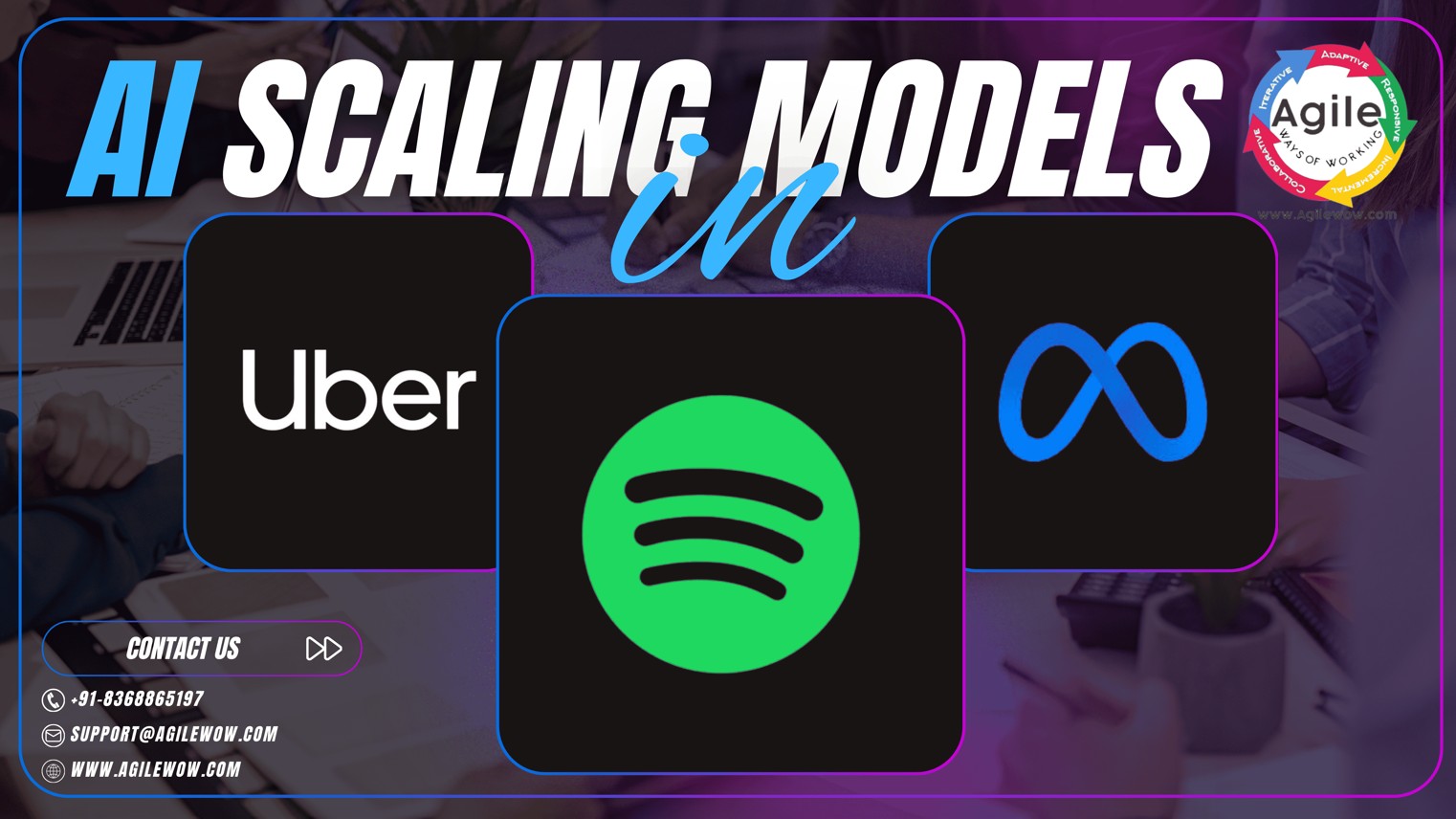
In the previous article, we covered how to set up and organize your AI teams and explored different scaling models. This article delves into real-world examples of each scaling model implementation by various organizations.
Different organizations have adopted distinct approaches to structuring and scaling their AI teams. Below are examples of companies across various industries and sizes, showcasing the models they've used and how their AI teams are organized.
1. Centralized AI Center of Excellence (CoE)
JPMorgan Chase – Centralized Machine Learning CoE
The bank established a Machine Learning Center of Excellence (MLCoE) as a central hub for AI specialists. This team of expert ML scientists collaborates closely with different business units, partnering to develop and deploy AI solutions across the enterprise.
By centralizing top AI talent in a CoE, JPMorgan ensures consistent standards and governance while aligning projects with diverse business needs such as banking, markets, and risk. An internal AI/ML Council provides oversight and facilitates the sharing of learnings across projects.
Walmart – Centralized AI CoE
The retail giant created an AI Center of Excellence with strong executive support to drive AI adoption throughout the company. This CoE is a cross-functional team comprising data scientists, engineers, business analysts, and domain experts from various departments.
This centralized unit collaborates with business units to identify relevant use cases and implement AI solutions, including personalization in e-commerce and supply chain optimization, ensuring alignment with strategic objectives. The centralized team also establishes standards for data infrastructure and governance and provides training to upskill employees in AI.
Siemens – Central AI Lab/CoE
Industrial manufacturer Siemens employs a centralized AI Lab as the focal point for its AI initiatives. Integrated within the Siemens ecosystem, the AI Lab drives industrial AI transformation by offering expert insights, training programs, and incubation for AI projects.
Functioning as a CoE, a small central team of AI experts works with various business units, such as factory automation, energy, and healthcare equipment, to pilot AI solutions and disseminate best practices. This model centralizes AI R&D while distributing knowledge and tools to engineering teams across the company.
--------------------------------------------------------------------------------------------------------------------------------
2. Decentralized (Embedded) AI Teams
Booking.com – Embedded Data Science Teams
The online travel company utilizes an embedded model for its data science teams. Data scientists are integrated directly into product teams rather than residing in a central group – an "embedded structure" where each product or business team has its own data scientist working alongside engineers and product managers.
This decentralized approach allows data scientists to concentrate on the specific needs of their product area, such as pricing, recommendations, or A/B experimentation for UX, and to drive AI solutions in close collaboration with domain teams. It fosters agility and deep business understanding, although it necessitates strong coordination to prevent isolated efforts.
Small Tech Firms / Startups – Decentralized by Necessity
Many smaller companies early in their AI journey also adopt decentralized models. Instead of a formal central AI unit, they directly hire data scientists into different teams or task software engineers with ML responsibilities. For instance, a small fintech startup might embed one data scientist in the fraud team and another in marketing analytics. This can accelerate initial AI feature development by keeping talent close to the problem; however, companies often introduce more central coordination as they scale to ensure consistent practices.
--------------------------------------------------------------------------------------------------------------------------------
3. Hybrid Model (Central Hub with Embedded Teams)
Airbnb – Hybrid Centralized/Embedded Data Science
Airbnb transitioned from a fully centralized data science team to a hybrid model as the company expanded. Initially, all data scientists were part of a central group, which helped maintain consistent methodologies but led to siloing. Airbnb then reorganized, maintaining the data science team as a single unit for career development and standards but dividing it into sub-teams aligned with product and functional areas.
In this hybrid structure, data scientists are embedded as proactive partners with engineers, designers, and product managers within their respective departments while still reporting to a central data science leadership. This approach preserves a "neural core" of shared knowledge across the company while ensuring each business unit has dedicated data science support for decision-making and product features.
Meta (Facebook) – Federated (Hybrid) Model
Facebook's data organization exemplifies a federated hybrid approach. Individual product teams at Facebook have end-to-end ownership of their data/AI projects—they decide on the problems to solve and build the solutions within their product domain. However, common infrastructure and tools are provided by central teams, creating an internal "AI platform" accessible to everyone.
For example, Facebook's centralized data engineering group standardizes tooling for data processing, experimentation, and dashboards, which all teams adopt. This hub-and-spoke model allows autonomy within each team (spoke) while a central hub ensures consistency in technology and best practices. It effectively represents a hybrid model: decentralized execution of AI projects supported by a central platform and governance.
--------------------------------------------------------------------------------------------------------------------------------
4. AI-as-a-Platform (Central Platform Team with Company-wide AI Enablement)
Uber – ML Platform (AI-as-a-Platform) + Embedded Specialists
Uber scaled its AI efforts by developing Michelangelo, a centralized machine learning platform team that offers ML-as-a-service to all other teams.
The Michelangelo platform team develops the necessary tools, frameworks, and infrastructure to enable Uber's diverse product teams (rides, Eats, mapping, etc.) to train, deploy, and operate ML models at scale. In Uber's model, product engineering teams own the models they build and deploy, staffing their teams with the required data scientists or ML engineers and utilizing the central platform for support.
When advanced expertise is needed, such as in NLP or computer vision, specialized AI teams and researchers step in to provide assistance. This AI-as-a-Platform approach, a form of hybrid, democratizes ML capabilities across the company. The platform team focuses on developer velocity and tooling, while each business unit can integrate AI features with end-to-end ownership.
Spotify – ML Platform Guild (Hybrid Platform)
The music streaming firm Spotify employs a variation of the platform model aligned with its "Squad/Tribe" organizational style. Spotify has a central ML platform/Research team that provides recommendation algorithms and ML infrastructure as a service, such as the team responsible for building Spotify's music recommendation models and Pipeline tooling. Simultaneously, product squads in areas like personalization, ads, or content include data scientists or ML engineers who leverage these platform services to build features. This platform-plus-embedded approach ensures both innovation (central R&D on cutting-edge algorithms) and rapid product integration (squads quickly applying AI to user-facing features).
--------------------------------------------------------------------------------------------------------------------------------
Each of these companies illustrates how different AI team structures can lead to success. The optimal model often depends on factors such as the company's size, industry, and AI maturity level. A centralized CoE offers control and shared expertise, which can be particularly beneficial in large or traditionally structured organizations. A decentralized/embedded model provides agility and strong business alignment, common in smaller or fast-paced product companies. Hybrid and platform-based models aim to combine the advantages of both by centralizing knowledge and tools while decentralizing day-to-day AI development for speed and context. Ultimately, successful companies adapt their approach as they scale, sometimes starting with a centralized model to build core capabilities and later federating the team structure to meet the increasing demand for AI across the organization.
Sources:
JPMorgan: https://www.technologyreview.com/2023/06/12/1074272/successfully-deploying-machine-learning/
Booking.com: https://medium.com/data-science/diary-of-a-data-scientist-at-booking-com-924734c71417
AirBnB: https://medium.com/airbnb-engineering/at-airbnb-data-science-belongs-everywhere-917250c6beba
Uber: https://www.uber.com/en-IN/blog/scaling-michelangelo/