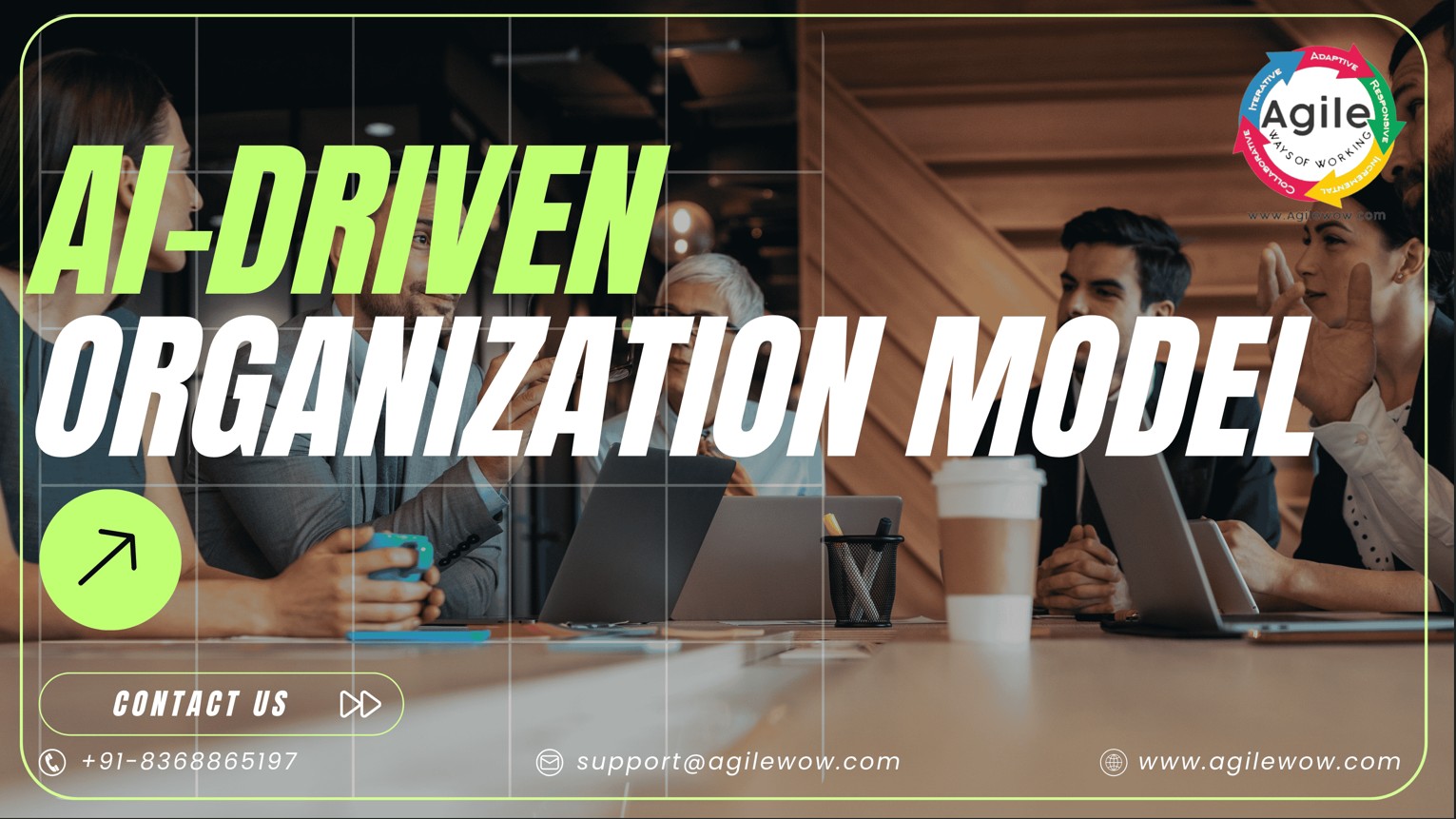
To succeed with AI transformation, organizations need structures specifically designed for the unique demands of AI development, deployment, and innovation. Traditional hierarchical structures and siloed functional groups often hinder AI initiatives due to slow decision-making and limited adaptability.
Drawing from experience in scaling agile practices in innovative, technology-intensive environments, here's an organizational structure optimised explicitly for AI initiatives:
Core Principles for AI-Driven Organizations
- Data-Driven Autonomy: Teams empowered with autonomy, guided by data insights and continuous experimentation.
- Rapid Learning Cycles: Short, iterative cycles of AI model experimentation, testing, and learning.
- Cross-functional collaboration: Combining AI specialists, data professionals, business stakeholders, and domain experts in tightly integrated teams.
- Continuous Innovation: Structured to facilitate innovation through community-driven knowledge sharing and experimentation.
AI-Optimized Team Structures
1. AI Pods (Cross-Functional AI Teams)
Similar to small agile teams but explicitly optimised for AI workloads:
- Machine Learning Engineers
- Data Scientists
- Data Engineers
- AI Product Managers
- Domain/Business Experts
- UX/UI Designers (when relevant)
- MLOps Specialists
Responsibilities:
- Own end-to-end AI development cycles: from ideation, data exploration, and model prototyping to deployment and monitoring.
- Conduct rapid, iterative experiments (A/B tests, quick pilots, MVP deployments).
Continuously optimize AI models and solutions based on real-time user and data feedback.
2. AI Domains (Strategic Groups)
Groups of AI Pods focused around key strategic business domains or product areas:
Examples:
- Customer Engagement & Personalization
- Supply Chain & Logistics Optimization
- Predictive Analytics and Decision Support
- AI-driven Product Innovation & R&D
Responsibilities:
- Ensure the strategic alignment of AI Pods with the organizational vision and business outcomes.
- Facilitate shared resources and strategic direction to maximize AI initiatives' impact.
Promote inter-Pod collaboration within the domain for optimal resource use and strategic coherence.
3. AI Capability Networks (Skill Networks)
Organized around specific AI expertise and disciplines, these communities span across AI Pods and Domains to promote knowledge sharing and functional excellence:
Examples:
- Natural Language Processing (NLP) Capability Network
- Computer Vision and Image Processing Network
- MLOps & AI Infrastructure Network
- AI Ethics & Responsible AI Network
Responsibilities:
- Provide specialized expertise, training, and continuous skill development to ensure that organizational AI capabilities grow continuously.
- Maintain AI best practices, standards, and consistency across Pods and Domains.
Actively facilitate cross-team knowledge sharing, ensuring rapid transfer of technical breakthroughs.
4. AI Innovation Labs (Knowledge Communities)
These informal groups encourage innovation, experimentation, and continuous learning across the entire organization, breaking down silos and fostering collaboration around emerging AI technologies and trends.
Examples:
- Generative AI Innovation Lab
- AI-driven Automation Lab
- AI Product Design and Innovation Lab
Responsibilities:
- Promote bottom-up innovation through regular hackathons, tech talks, research sharing, and experimental prototyping.
- Continuously evaluate and integrate new AI technologies and methods into organizational practices.
Drive exploratory AI projects that may lead to breakthrough innovations.
5. AI Governance & Leadership
Central AI Steering Group
This strategic group coordinates the overall AI vision, strategy, and governance. It ensures alignment, resource allocation, and effective prioritization across all AI Pods and Domains.
Responsibilities:
- Set the organizational AI strategy and priorities based on strategic business objectives.
- Oversee ethical AI practices and responsible governance (transparency, fairness, bias mitigation).
- Provide strategic resources (talent, funding, technology infrastructure) and address structural challenges proactively.
Role of Chief AI Officer (CAIO)
- A dedicated executive responsible for driving AI initiatives across the organization:
- Advocates strategic direction, clears barriers, and aligns stakeholders.
- Encourages an agile, iterative approach to AI innovation.
Acts as the link between executive leadership and the AI organization.
Why this AI Org Model works:
- Rapid adaptability: Enables swift pivots as AI projects evolve, preventing stagnation or misalignment.
- Continuous experimentation: AI Pods use iterative cycles to test ideas, accelerating innovation and reducing risk.
- Specialized expertise & knowledge sharing: Capability Networks ensure best practices and skills continuously evolve and disseminate.
- Alignment without rigidity: AI Domains and the Steering Group ensure teams stay aligned with strategic goals, while still maintaining local autonomy for innovation.
Implementing an AI transformation demands rethinking traditional organizational structures. Successful AI teams require autonomy, flexibility, iterative practices, and extensive cross-functional collaboration.
This AI-driven organizational model balances strategic coherence, rapid experimentation, and continuous learning, setting the foundation for successful, scalable AI transformations.
This blog was originally published on AgileWoW